You are welcome to bring along a poster.
Workshop on Themes across Control and Reinforcement Learning
Following our Spring School 2025 of the research semester programme on Control Theory and Reinforcement Learning, we have a general workshop on Themes across Control and Reinforcement Learning.
Speakers information
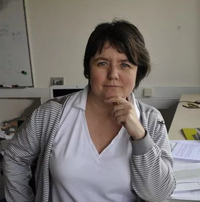
Ann Nowé graduated from the University of Ghent in 1987, where she studied mathematics with optional courses in computer science. She then became research assistant at the University of Brussels where she finished her PhD in 1994 in collaboration with Queen Mary and Westfield College, University of London. The subject of her PhD is located in the intersection of Computer Science (A.I.), Control Theory (Fuzzy Control) and Mathematics (Numerical Analysis, Stochastic Approximation). After a period of 3 years as senior research assistant at the Vrije Universiteit Brussel (VUB), she became a Postdoctoral Fellow of the Fund for Scientific Research-Flanders (F.W.O.). Nowadays, She is a professor both in the Computer Science Department of the faculty of Sciences as in the Computer Science group of the Engineering Faculty. She coordinates the project CTRLXAI, on automated controller generation at the intersection of control engineering and machine learning.
Talk details
When RL and Control meet: lessons learned
In this talk, I will share some lessons learned from collaborating on control problems within interdisciplinary teams. These insights will be framed around aspects such as use case selection, acceptance, and guarantees.
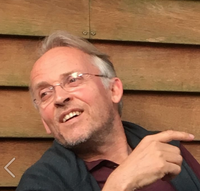
Bert Kappen completed his PhD in theoretical particle physics in 1987 at the Rockefeller University in New York. From 1987 until 1989 he worked as a scientist at the Philips Research Laboratories in Eindhoven, the Netherlands. Since 2004 he is full professor on machine learning and neural networks at the science faculty of the Radboud University. In 1998, he co-founded the company Smart Research that commercializes applications of neural networks and machine learning.
Bert Kappen conducts research on neural networks, Bayesian machine learning, stochastic control theory and computational neuroscience. Currently, he is investigating ways to use quantum mechanics for a new generation of quantum machine learning algorithms and control methods for quantum computing.
Talk details
Title: Path integral control for open quantum systems
We consider the generic problem of state preparation for open quantum systems. As is well known, open quantum systems can be simulated by quantum trajectories described by a stochastic Schr\"odinger equation. In this context, the state preparation problem becomes a stochastic optimal control (SOC) problem. The SOC problem requires the solution of the Hamilton-Jacobi-Bellman equation, which is generally challenging to solve. A notable exception are the so-called path integral (PI) control problems for which one can estimate the optimal control solution by sampling. We derive a class of quantum state preparation problems that can be solved with PI control. Since our method only requires the propagation of state vectors $\psi$, it presenting a quadratic advantage over density-centered approaches, such as PMP. Unlike most conventional quantum control algorithms, it does not require computing gradients of the cost function to determine the optimal controls. We illustrate the practicality of our algorithm through multiple examples for single- and multi-qubit systems.
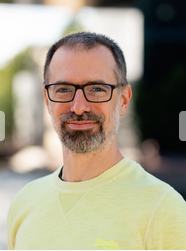
Davide Grossi is an associate professor (adjunct hoogleraar) in Multi-Agent Decision Making, affiliated to the Bernoulli Institute for Mathematics, Computer Science and Artificial Intelligence at the University of Groningen . He is a member of the Multi-Agent Systems Group, and of the Groningen Cognitive Systems and Materials Center (CogniGron).
At the University of Amsterdam he is an associate professor affiliated to the Amsterdam Center for Law and Economics (ACLE), and the Institute for Logic, Language and Computation (ILLC).
Talk details
Title: Learning to Cooperate under Uncertain Incentive Alignment
We apply a multi-agent reinforcement learning approach to study the emergence of cooperation among reinforcement learning agents that interact in a game. Crucially, agents are however uncertain about the extent to which their incentives are aligned, or misaligned, in the game. In this framework, we explore through computational experiments under what conditions further features of the model (like communication, reputation mechanisms, varying risk attitudes) may support learning of more cooperative policies.
This is joint work with: Nicole Orzan (University of Groningen), Erman Acar (University of Amsterdam) and Roxana Radulescu (Utrecht University)
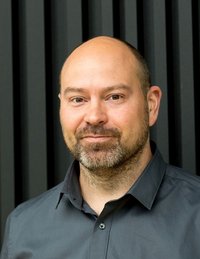
Frans A. Oliehoek is Associate Professor at Delft University of Technology, where he is a leader of the sequential decision making group, a scientific director of the Mercury machine learning lab, and director and co-founder of the ELLIS Unit Delft. He received his Ph.D. in Computer Science (2010) from the University of Amsterdam (UvA), and held positions at various universities including MIT, Maastricht University and the University of Liverpool. Frans' research interests revolve around intelligent systems that learn about their environment via interaction, building on techniques from machine learning, AI and game theory. He has served as PC/SPC/AC at top-tier venues in AI and machine learning, and currently serves as associate editor for JAIR and AIJ. He is a Senior Member of AAAI, and was awarded a number of personal research grants, including a prestigious ERC Starting Grant.
Talk details
Title: Learning and using models for controlling complex environments
In reinforcement learning (RL), we develop techniques to learn to control complex systems, and over the last decade we have seen impressive successes ranging from beating grand masters in the game of Go, to real-world applications like chip design, power grid control, and drug design. However, nearly all applications of RL require access to an accurate and lightweight model or simulator from which huge numbers of trials can be sampled. In this talk, I will discuss some topics in model-based RL, in which this is not the case, but where we instead try to learn such models.
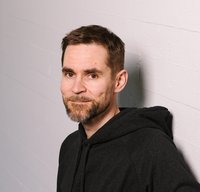
Harri Lähdesmäki is an Associate Professor (tenured) in Department of Computer Science at Aalto University, where he leads the Computational Systems Biology research group. He works on probabilistic machine learning with applications in biomedicine and molecular biology. His current research interests include deep generative models, dynamical systems, longitudinal analysis, single-cell biology, and health applications.
Talk details
Title: Learning latent dynamics models from complex and high-dimensional data
There is an abundance of dynamical systems around us, including physical systems, biological networks, industrial processes, population dynamics, and social graphs, which we would like to model and control. While models in some applications can be derived analytically, there are many systems whose governing equations cannot be derived from the first principles because their behavior is too complex and poorly understood or dimensionality far too high. In this talk, I will present our recent work on developing methods to learn latent continuous-time dynamics models from complex and high-dimensional (spatio)temporal data. Our approach builds on formulating these models as neural net parameterized generative differential equation systems that can be learned using efficient amortized variational inference methods and used for long-term predictions as well control approaches.
This is joint work with Valerii Iakovlev, Cagatay Yildiz, and Markus Heinonen.
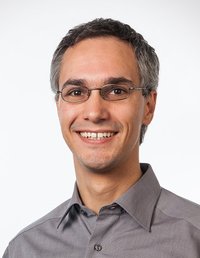
Jens Kober is an associate professor at TU Delft, Netherlands. He is member of the Cognitive Robotics department (CoR) and the TU Delft Robotics Institute.
Jens is the recipient of the Robotics: Science and Systems Early Career Award 2022 and the IEEE-RAS Early Academic Career Award in Robotics and Automation 2018. His Ph.D. thesis has won the 2013 Georges Giralt PhD Award as the best Robotics PhD thesis in Europe in 2012.
Talk details
Reinforcement Learning for Robot Control
Reinforcement Learning has emerged as one of the most prominent paradigms to enable advanced robot control. A prime example is locomotion of quadrupeds over challenging terrains, where policies learned through RL are now being shipped with commercial robot platforms. Nevertheless, robot RL faces specific challenges as due to their physical embodiment collecting large amounts of real-world interaction data is impossible. To render robot reinforcement learning tractable, prior information can be integrated in various ways, ranging from simulations (sim2real), human demonstrations or corrections, to generative models (e.g., LLMs).
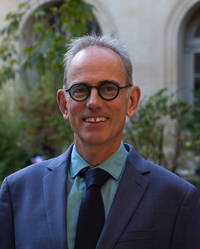
Sean Meyn was raised by the beach in Southern California. Following his BA in mathematics at UCLA, he moved on to pursue a PhD with Peter Caines at McGill University. After about 20 years as a professor of ECE at the University of Illinois, in 2012 he moved to beautiful Gainesville. He is now Professor and Robert C. Pittman Eminent Scholar Chair in the Department of Electrical and Computer Engineering at the University of Florida, and director of the Laboratory for Cognition and Control. He also holds an Inria International Chair to support research with colleagues in France. His interests span many aspects of stochastic control, stochastic processes, information theory, and optimization. For the past decade, his applied research has focused on engineering, markets, and policy in energy systems.
Talk details
The Projected Bellman Equation in Reinforcement Learning
A topic of discussion throughout the 2020 Simons program on reinforcement learning: is the Q-learning algorithm convergent outside of the tabular setting? It is now known that stability can be assured using a matrix gain algorithm, but this requires assumptions, which begs the next question: does a solution to the projected Bellman equation exist? This is the most minimal requirement for convergence of any algorithm.
The question was resolved in very recent work. A solution does exist, subject to two assumptions: the function class is linear, and (far more crucial) the input used for training is a form of epsilon-greedy policy with sufficiently small epsilon. Moreover, under these conditions it is shown that the Q-learning algorithm is stable, in terms of bounded parameter estimates. Convergence remains one of many open topics for research.
In short, sufficient optimism is not only valuable for algorithmic efficiency, but is a means to algorithmic stability.
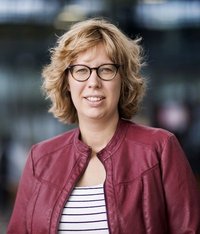
Sofie Haesaert is an Associate Professor in the Control Systems group of the Department of Electrical Engineering at Eindhoven University of Technology. Her research interests lie in the development of verification and control synthesis methods for cyber-physical systems. She holds a bachelor's degree in Mechanical Engineering and a master's degree in Systems and Control from Delft University of Technology, both cum laude. As a master's degree student, Haesaert spent a summer doing research at the University of Texas Arlington (USA). Haesaert obtained her PhD from Eindhoven University of Technology (TU/e) in 2017 and then went to Caltech (USA) for 17 months to do a postdoc. In 2018, Haesaert returned to TU/e to become Assistant Professor in the Control Systems group.
Talk details
Using data to tackle uncertainty in correct-by-design control synthesis
We often lack exact model knowledge of the dynamics of systems that we want to control in a verifiable manner. This can be due to lack of information on the physical composition of dynamic systems and its environment or due to wear and tear of the physical system during operation. Using data-driven approaches, controllers for these systems can be still formally verified. In this talk I will cover some of our results on data-driven verification and control synthesis for linear and nonlinear (stochastic) dynamics. I will talk about direct data-driven control synthesis and about Bayesian data-driven and model-based approaches.
Programme
09:15 - 09:50 Registration and tea/coffee
09:50 - 10:00 Welcome
10:00 - 11:00 Sean Meyn, The Projected Bellman Equation in Reinforcement Learning (slides, video)
11:00 - 11:30 Break
11:30 - 12:30 Contributed talks
Caio Kalil Lauand, Sean Meyn: The Curse of Memory in Stochastic Approximation
Simon Gottschalk, Hierarchical Control Strategies Based on a Reinforcement Learning Planner
Stephan Bongers, Onno Zoeter, Matthijs T.J. Spaan and Frans A. Oliehoek: Anytime-valid off-policy evaluation for reinforcement learning
Stavros Orfanoudakis, Nanda Kishor Panda, Peter Palensky, Pedro P. Vergara: GNN-DT: Graph Neural Network Enhanced Decision Transformer for Efficient Optimization in Dynamic Environments
12:30 - 14:00 Lunch and Poster session
14:00 - 14:40 Jens Kober, Reinforcement Learning for Robot Control (slides, video)
14:40 - 15:20 Sofie Haesaert, Using data to tackle uncertainty in correct-by-design control synthesis
15:20 - 15:50 Break
15:50 - 16:30 Bert Kappen, Path integral control for open quantum systems (slides, video)
16:30 - 18:00 Posters and Discussion with drinks and bites
09:30 - 10:00 Registration and tea/coffee
10:00 - 11:00 Ann Nowé, When RL and Control meet: lessons learned (slides, video)
11:00 - 11:30 Break
11:30 - 12:30 Contributed talks
Reabetswe Nkhumise, Debabrota Basu, Tony Prescott, Aditya Gilra: Studying exploration in RL: an optimal transport analysis of occupancy measure trajectories
Gabriel Nova, Sander van Cranenburgh, Stephane Hess: Improving choice model specification using reinforcement learning
Zhao Yang, Thomas M. Moerland, Mike Preuss, Aske Plaat, Edward S. Hu: Reset-free Reinforcement Learning with World Models
Safwan Labbi, Daniil Tiapkin, Lorenzo Mancini, Paul Mangold, Eric Moulines: Federated UCBVI: Communication-Efficient Federated Regret Minimization with Heterogeneous Agents
12:30 - 14:00 Lunch and Poster session
14:00 - 15:00 Harri Lähdesmäki, Learning latent dynamics models from complex and high-dimensional data
15:00 - 15:30 Break
15:30 - 16:10 Frans Oliehoek, Learning and using models for controlling complex environments (slides, video)
16:10 - 16:50: Davide Grossi, Learning to Cooperate under Uncertain Incentive Alignment (slides, video)
16:50 - 18:00 Posters and Discussion with drinks
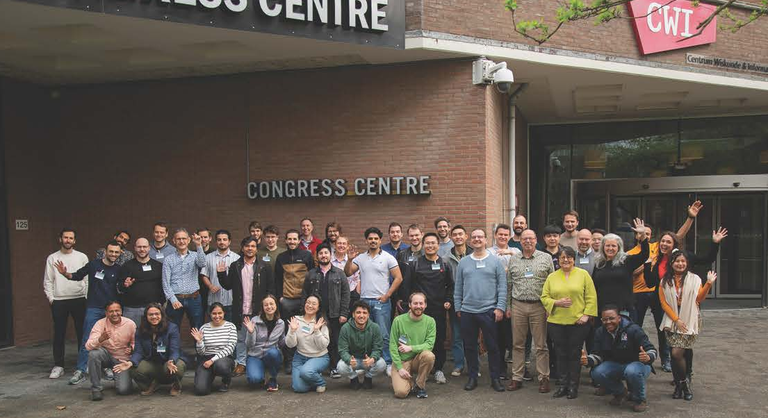
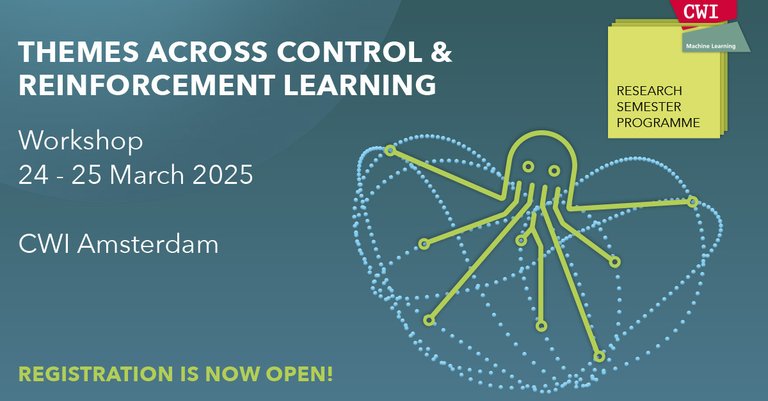
Please click here to download and print the poster, to spread within your network.
Click the link for more information about the Research Semester Programme "Control Theory and Reinforcement Learning: Connections and Challenges".