The first annual meeting of the Dutch Inverse Problems Community will take place on 25-26 November 2021 in conference center de Werelt, Lunteren.
Program:
Thursday 25 November
09.30 - 10.00: Reception, coffee
10.00 - 12.30: Masterclasses 1 & 2 (in parallel)
12.30 - 14.00: Lunch break
14.00 - 16.30: Masterclasses 1 & 2 (in parallel)
16.30 - 18.00: Drinks and brainstorm session
18.30 - 20:00 : Dinner
Friday 26 November
08.45 - 09.15: Reception, coffee
09.15 - 9.30: Opening
09.30 - 10.30: Hai Xiang Lin: Data assimilation and machine learning for air quality forecasts
Rikkert Hindriks: Reconstruction of functional brain networks from EEG/MEG sensor data.
10.30 - 11.00: Coffee break
11.00 - 12.00: Maureen van Eijnatten: Deep learning for image-guided treatments
Jeannot Trampert: Estimating the posterior using prior sampling: The case of rapid seismic source inference.
12.00 - 13.30: Lunch break
13.30 - 14.30: Harry van Zanten: Bayesian inverse problems
Jeroen Kalkman: Imaging challenges in optical tomography.
14.30 - 16.30: Panel discussion
16:30 - 17.30: Drinks
Masterclasses
1. Optimisation techniques in inverse problems - Juan Peypouquet In this course, we will discuss how some optimisation techniques can be used to analyse and solve a class of inverse problems. The course will contain a self-contained review of convex analysis, subdifferential calculus and optimality conditions, plus an introduction to iterative methods used to solve these kinds of problems. Entry requirements: Bachelor-level knowledge of functional analysis and differential calculus is recommended
2. Data assimilation - Femke Vossepoel Data assimilation combines dynamic models with available observations to find the probability distribution of the model solution given the data. In the last decades, we see a growing application of data assimilation in the geosciences, but also in other fields, from economics to epidemiology. In this master class, we will explain the principles of data assimilation from a Bayesian perspective and provide a unified formulation of data assimilation that places various data assimilation methods and their applications in perspective. We will discuss how to use data assimilation for state and parameter estimation and we will discuss how these methods can deal with errors in the dynamic model and its control or forcing. Participants will experience the possibilities and limitations of data assimilation in an exercise with a toy problem. Entry requirements: MSc-level education in physics, maths, engineering or geosciences. Basic knowledge of inverse problem theory and Bayesian statistics.
Lectures
Hai Xiang Lin - Data assimilation and machine learning for air quality forecasts. Atmospheric models contain many uncertainties in initial and boundary values, parameters and chemical and physical processes not fully modelled in the dynamic equations, these uncertainties lead to inaccurate forecasts. Data assimilation (DA) and machine learning (ML) techniques can be used to improve the models with the information from the observations. Several research projects on improving the prediction accuracy of volcanic ash clouds during eruption and PM2.5 and PM10 concentrations in dust storms will be discussed. Typically, due to limited measurement on the emission site, there are great uncertainties about the erupted amount of volcanic ashes and the dust emission from the desert. DA and ML methods are applied to perform emission inversions.
Rikkert Hindriks - Reconstruction of functional brain networks from EEG/MEG sensor data. Electroencephalography (EEG) and magnetoencephalography (MEG), respectively, record scalp potentials and magnetic fluxes surrounding the head that are induced by electrical currents inside the brain. I will discuss the biophysical principles underlying such measurements and provide an overview of the inverse methods that are used to reconstruct brain activity from sensor EEG/MEG data. I will focus on the reconstruction of functional brain networks, which is currently one of the most activity areas of research in this field.
Maureen van Eijnatten - Deep learning for image-guided treatments. Deep learning has enormous potential to automate and augment various image-guided treatments, thereby opening completely new avenues to personalize patient care. However, there are still some challenges to overcome when applying state-of-the-art deep neural networks to medical images. Training such networks, for example, requires vast amounts of (imaging) data including accurate ground truth labels that typically need to be manually created, whereas increasingly strict privacy regulations prohibit sharing patient data between institutions. Furthermore, existing deep learning methods often do not generalize well to variations in imaging protocols, scanner manufacturers and patient populations. This lecture will highlight some of the main opportunities and challenges of deep learning in the areas of maxillofacial surgery, oncologic surgery and radiotherapy.
Jeannot Trampert - Estimating the posterior using prior sampling: The case of rapid seismic source inference. Suppose that we have a non-linear problem d=g(m), where d is a data vector, m the model vector to be inferred and g the mapping. In the Bayesian framework, the problem consists of estimating the probability density function p(d|m). In the absence of a closed form answer, the problem can be solved by sampling. I will show that this sampling can be approached in two ways, by posterior sampling (e.g. MCMC) or prior sampling. The latter can be numerically advantageous when an inference problem must be solved fast or repeatedly. The main difference between the two sampling strategies is at what stage you introduce the actual measurements. Just as there are many algorithms for posterior sampling, the inference based on prior samples can be done in various ways. We chose mixture density networks (MDNs). I will quickly explain the principle before moving to a toy example illustrating the main points. I will then show a real example for the rapid inference of moment tensors in the Californian region.
Refs:
Kaeufl P., Valentine A.P., de Wit R.W.L., Trampert J., 2016. Solving probabilistic inverse problems rapidly with prior samples, Geophys. J. Int., 205, 1710–1728.
Kaeufl P., Valentine A.P., Trampert J., 2016. Probabilistic point source inversion of strong-motion data in 3-D media using pattern recognition—a case study for the 2008 Mw 5.4 Chino Hills earthquake, Geophys. Res. Lett., 43, doi:10.1002/2016GL069887.
Harry van Zanten - Bayesian inverse problems. In recent years the Bayesian approach to solving inverse problems has become quite popular. In this talk I intend to give a brief overview of some of the mathematical work that has been done on the behavior and performance of these methods.
Jeroen Kalkman - Imaging challenges in optical tomography. In optical tomography light does not behave like straight pencil-like rays that X-rays do in X-ray computed tomography. Light propagation is strongly influence by diffraction, refraction, and scattering. When not properly taken into account in the system design and reconstruction algorithms these effects can severely affect the image quality in optical tomography. In this presentation I will show how some of these effects can be mitigated in hardware by applying optical sample clearing or physical measurement principles such as coherent and confocal gating. I will show how the effects of diffraction on the reconstructed image have been addressed through analytical theory of the optical point spread function combined with 2D image deconvolution in the reconstructed image. In addition, we have dealt with these effects through iterative reconstruction of the inverse problem discretising the object and the imaging geometry. We have solved the inverse problem in 2D, however challenges remain to solve the optical tomography diffraction in 3D for an extremely large data volume (>109 voxels).
Acknowledgements
The meeting was financially supported by NDNS+, Marie Skłodowska-Curie Research and Innovation Staff Exchange action (MSCA-RISE) No. 777826 "Nonlocal Methods for Arbitrary Data Sources (NoMADS)" and the Delphi consortium.
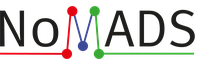
