- Dick den Hertog (University of Amsterdam)
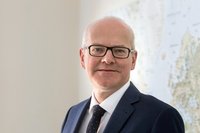
Analytics for a better world - presentation
abstract:
In this talk I will describe two Analytics applications that contribute to one or more of the 17 Sustainable Development Goals (SDGs) of the United Nations. The first application is an optimization model to optimize the food supply chain for the World Food Programme. This application received the Franz Edelman award in 2021. The second application is an optimization model to optimize health care facility locations in Timor-Leste and stroke centers in Vietnam. This project is carried out in collaboration with the World Bank. We show that uncertainty plays an important role in such practical problems, and we argue that Robust Optimization is an effective methodology to obtain robust solutions. We finally discuss the new movement 'Analytics for a Better World', which was recently initiated by Dimitris Bertsimas (MIT) and the speaker.
bio:
Dick den Hertog is professor of Operations Research at University of Amsterdam. His research interests cover linear and nonlinear optimization. In recent years his main focus has been on robust optimization, and recently he started research on optimization with constraint learning. He is also active in applying the theory in real-life applications. In particular, he is interested in applications that contribute to a better society. For many years he has been involved in research for optimal flood protection, which was awarded by the INFORMS Franz Edelman Award in 2013. He is involved in research to optimize the food supply chain for the UN World Food Programme, which was awarded by the INFORMS Franz Edelman Award in 2021. He is associate editor of "Operations Research" and "INFORMS Journal on Optimization", and editor of "Analytics for a Better World". He has been visiting professor at MIT since 2019.
- Pinar Keskinocak (Georgia Institute of Technology)
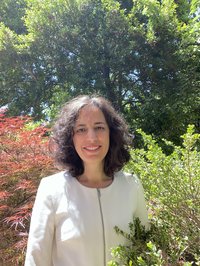
Modeling Infectious Diseases: Projecting Spread, Evaluating Interventions, and Resource Allocation - presentation
abstract:
Our research team at Georgia Tech has worked on modeling a number of infectious diseases over the years, including pandemic flu, cholera, malaria, polio, Guinea worm, and Covid-19. We utilized different modeling approaches, such as SEIR, agent-based, integer programming, depending on the research questions or decision-support needs in practice. This presentation will provide an overview of some of our work in infectious disease modeling, and briefly discuss some dashboards we developed.
bio:
Pinar Keskinocak is the William W. George Professor and Chair in the School of Industrial and Systems Engineering at Georgia Tech and an Adjunct Professor in the Department of Environmental Health, Rollins School of Public Health at Emory University. She is the co-founder and Director of the Center for Health and Humanitarian Systems at Georgia Tech. Her main research areas include infectious disease modeling (including Covid-19, malaria, Guinea worm, pandemic flu, polio), evaluating intervention strategies, and resource distribution; process improvement for healthcare delivery; disaster preparedness, response, recovery; logistic and supply chain management. She has collaborated on projects with companies, governmental and non-governmental organizations, and healthcare providers, including American Red Cross, CARE, Carter Center, CDC, Children’s Healthcare of Atlanta, Emory University, and Task Force for Global Health.
She is an INFORMS Fellow and served as the 2020 president of INFORMS. She has also served on the editorial boards of several journals.
- Gergely Neu (Universitat Pompeu Fabra Barcelona)
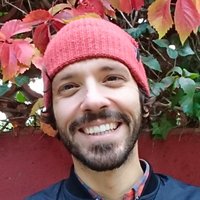
Reinforcement Learning via Linear Programming - presentation
abstract:
Reinforcement Learning (RL) is one of the leading frameworks for sequential decision-making under uncertainty, underlying many of the recent breakthroughs of artificial intelligence research such as
achieving human-level performance in the game of Go or Starcraft. Most modern RL methods are based on solving a high-dimensional system of nonlinear fixed-point equations called the Bellman equations, first formulated in the 1950s in the context of optimal control by Richard Bellman. Despite having inspired decades of productive work in
reinforcement learning, methods based on approximate solutions of the Bellman equations remain challenging to formally analyze due to the conceptual difficulty of working with nonlinear fixed-point equations.
In this talk, I highlight an equivalent alternative formulation of sequential decision making based on the conceptually simpler framework of Linear Programming (LP). While this formulation has been proposed as early as the 1960s by Alan Manne, it has received considerably less attention in the RL literature until recently, when it has finally started to gain popularity due to its simplicity and elegance. This talk aims to present some of the numerous recent advances rooted in the LP framework, highlighting how the key limitations associated with past LP-based approaches have been eliminated and how the LP perspective enabled a deeper formal understanding of empirically successful RL methods.
bio:
Gergely Neu is a research assistant professor at the Pompeu Fabra University, Barcelona, Spain. He has previously worked with the SequeL team of INRIA Lille, France and the RLAI group at the University of Alberta, Edmonton, Canada. He obtained his PhD degree in 2013 from the Budapest University of Technology and Economics, where his advisors were András György, Csaba Szepesvári and László Györfi. His main research interests are in machine learning theory, including reinforcement learning and online learning with limited feedback and/or very large action sets. Dr. Neu was the recipient of a Google Faculty Research award in 2018, the Bosch Young AI Researcher Award in 2019, and an ERC Starting Grant in 2020.
- Leonard Smith (Virginia Tech)
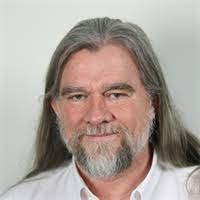
Opacity and Insight of Science in Support of Decision Making - presentation
abstract:
Decision making under uncertainty is hampered both by different varieties of uncertainty and by the wide variety of situations in which decisions are made. This presentation will focus on the role of uncertainty due to opacity; imprecision, indeterminacy, ambiguity and intractability will also come into play. Opacity captures uncertainty due to a lack of clarity on assumptions made within or to a failure in understanding the strength of scientific evidence. Provision of quantitative details from multiple simulation models or many forecasters can either increase of decrease opacity.
Opacity is increased when statistical manipulations are hidden; this is the case regardless of their rigour. It decreases when assumptions are made clear, again regardless of their rigour. There are, of course, Machiavellian attempts to increase opacity; a past lack of transparency can prove costly here. That said, there are also significant opportunities to advance the understanding of science by clarifying obfuscation, whether intentional or due to ignorance. Opacity is decreased when the limitations of scientific insight are made clear at the outset, when the source and potential impacts of each challenge are conveyed, and a timetable for lifting each is given.
All science is uncertain. The personal risk and public commitment of a decision maker can be impacted significantly by their confidence in their own understanding of the strengths and the weaknesses of the scientific evidence. It is suggested that confidence within the current level of uncertainty(s) is more robust when those uncertainties are grasped, leading to better decision making, and to a clearer vision on the part of decision makers as to evidence will be most value to them on the timescales of interest to them.
bio:
My group has been active in the formation, interpretation, evaluation and attempted application of probability forecasts in all areas, with a general interest in improving simulation modelling and scientific support for decision making, and aspirations of relevant uncertainty quantification in the escape from model land. We work directly with policy makers and practitioners in energy, banking, (re)insurance and disaster risk reduction, as well as developing the more mathematical aspects of scientific evidence in support of decisions under uncertainty.
Leonard Smith is a Professor of Engineering at Virginia Tech in SPACE@VT. Previously he directed the LSE’s Centre for the Analysis of Time Series, and was a Senior Research Fellow (mathematics) at Pembroke College, Oxford. He holds the Royal Meteorological Society’s Fitzroy Prize , a Selby Fellowship from the Australian Academy of Sciences, and is an AGU Charney Lecturer. His book A Very Short Introduction to Chaos is available in eight languages. He tweets as @lynyrdsmyth.
Back to the main programme of the CWI Lectures 2021.