Wouter Edeling
Full Name
Dr. W.N. Edeling
Email
Phone
+31 20 592 4161
Room
L124
Department(s)
Function(s)
Scientific Staff Member, Chair Works Council
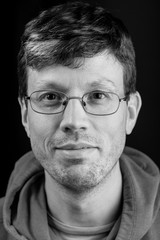
Publications
All publicationsAwards
-
Stanford university postdoctoral fellowship at the Center for Turbulence Research, 2015-2017. (2015)
Professional activities
-
Organizer: Nonlinear Dynamics of Natural Systems (NDNS+) workshop co-organizer 2021.
-
Organizer: Organizer mini-symposium SIAM UQ22
-
Speaker: Invited lecture on Uncertainty Quantification at the von Karman institute 2018.
-
Speaker: SIAM UQ18 , Los Angeles.
-
Showcased: Nature news: Adam, D., Simulating the pandemic: What COVID forecasters can learn from climate models, https://www.nature.com/articles/d41586-020-03208-1
-
Showcased: UK science museum: Highfield, R. CORONAVIRUS: VIRTUAL PANDEMICS , https://www.sciencemuseumgroup.org.uk/blog/coronavirus-virtual-pandemics/
-
Organizer: International conference for computational science (ICCS) thematic track organizer in 2020,2021 and 2022.
-
Showcased: Nature news & views : Leung, K., Wu, J.T., Quantifying the uncertainty of CovidSim https://www.nature.com/articles/s43588-021-00031-0
-
Showcased: Folia: Hoebe, H., UvA’ers: Brits coronarekenmodel gebrekkig, aantal doden kan vier keer zo hoog zijn https://www.folia.nl/wetenschap/144554/uvaers-brits-coronarekenmodel-gebrekkig-aantal-doden-kan-vier-keer-zo-hoog-zijn
-
Organizer: Co-organizer "The Role of Uncertainty in Mathematical Modeling of Pandemics” at the Isaac Newton Institute in Cambridge
-
Organizer: Co-organizer “Multiscale Modelling, Uncertainty Quantification and the Reliability of Computer Simulations” online conference, 2022
-
Speaker: 3 talks at Isaac Newton Institute (Cambridge U.): i) The Impact of Uncertainty on the CovidSim Pandemic Code, ii) Tutorial 1 EasyVVUQ, iii) Very Large Parameter Problems.
-
Speaker: Invited talk SCS spring meeting: High-dimensional parametric uncertainty quantification, Leuven, 2022.
-
Speaker: ECCOMAS : Learning reduced subgrid-scale models, Oslo, 2022.
-
Speaker: SIAM UQ 22, Reduced Data-Driven Parameterizations for Turbulent Flow, Atlanta.
-
Speaker: Invited talk CASA Day (Centre for Analysis, Scientific Computing and Applications, TU Eindhoven), April 2023.
-
Organizer: Organizer mini-symposium SIAM CSE 23
Current projects with external funding
-
Learning small closure models for large multiscale problems. (None)
Courses
-
Uncertainty Quantification(1 Sep 2023 - 9 Oct 2023)