In 2005, John Ioannidis, a professor at Stanford University, published a paper titled Why Most Published Research Findings are False. This has since led to an increased awareness that numerous research papers, even those in prestigious journals, may contain false-positive conclusions. This phenomenon has become known as the ‘replication crisis’.
Whether experimental results are significant and did not arise by chance is traditionally determined with p-values, using a method from the 1930s. “However, p-values are inappropriate for the way science is done today”, says Peter Grünwald, senior researcher at CWI. “P-values were invented for oneshot research. It is incorrect to add new data and recalculate the p-value, but that is what happens often in modern research. For example, if an experimental outcome of a drug trial is not significant, scientists often repeat the experiment by adding more participants. If one repeats this process, then, by pure chance, the result eventually will have a small p-value and look significant.”
Evidence-value
In recent years Grünwald and his CWI colleagues have developed a better alternative for the p-value, called E-value (evidence-value). Grünwald: “The E-value calculates how much evidence you have against the null hypothesis and is a value between zero and infinity. The higher the value, the higher the evidence that the outcomes are significant. In practice, in one-shot situations, significance is usually associated with a p-value smaller than 0.05. This corresponds to an E-value larger than 20, so you can say that if the E-value is larger than 20, the outcome can be accepted as significant. But you may now add data as long as you like, stop whenever you like and re-calculate the E-value, and still maintain the interpretation that an E-value larger than 20 means that the results are significant.”
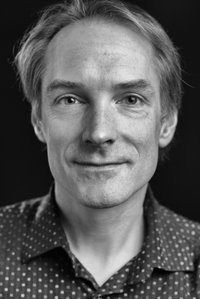
The concept of E-value dates back to the early 1970s, but was not embraced for decades. That changed in 2019. Suddenly the time appeared ripe and four articles by four different research groups appeared within six months, all arguing for the use of E-values in statistics and developing the underlying mathematics. It was Grünwald’s group at CWI that was the first and that also gave them a name.
“Our biggest contribution is that we have shown for the first time that there exists something like an optimal E-value”, says Grünwald. “Unfortunately, the optimal E-value is often difficult to calculate. Other groups have developed what may be called a quick-and-dirty E-value, but I mean this in a very positive sense. That one can be efficiently calculated, but is not optimal.”
Netflix and Amazon
In the world of theoretical statistics, E-values are beginning to gain acceptance. However, in the everyday practice of the rest of the scientific world, acceptance is proving difficult. Grünwald: “Millions of people worldwide are used to calculating p-values and software packages often have it integrated in their data analysis.”
Grünwald’s mission for the next few years is to promote E-values and also make their use easier by developing practical software. “The good news is that some major tech companies do already embrace E-values. About Netflix, I’m sure. About Amazon, I know they are working on it. The tech industry is more open to E-values because they have a huge interest in machine learning, and, interestingly, there are great similarities between E-value-based statistics and the fundamental problem of exploration versus exploitation in machine learning. Both are about being able to add new data at any time.”
The exploration-exploitation problem is a fundamental challenge in decision-making where one must choose between exploring new options and exploiting known options. Whenever a search engine has to choose which advertisements to show next to some search results it has to solve this problem.