CWI's SWAT and DIS group have been awarded with a prize at NWO's ICT OPEN 2019, the event for ICT research in the Netherlands.
The Software Analysis and Transformation group won the Best Software Engineering Technology Paper award for their article "Constraint-based Run-time State Migration for Live Modelling" derived from the Agile Language Engineering (ALE) team, together with their colleages from Inria, France.
The paper was presented at the VERSEN Software Engineering Track of ICT OPEN by Ulyana Tikhonova. This is the third paper winning an award resulting from the collaboration between CWI SWAT and INRIA Rennes in the context of the associate team ALE.
Authors: U. Tikhonova (Ulyana), T. van der Storm (Tijs), J.H. Stoel (Jouke) and T.F. Degueule (Thomas)
ABSTRACT
Live modeling is about improving the developer experience of domain-specific modeling languages by immediately reflecting changes to a
model into the running application, without restarting. A key problem is that the current run-time state of the application might become out of sync with the new version of the model. The paper introduces an approach to use constraint solving to obtain a new run-time state automatically. A new meta modeling language, called Nextep, allows language designers to declaratively specify the semantic relations between program model and run-time state, and their invariants, including rules for migration.
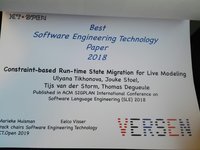
The Distributed and Interactive Systems group won a second prize for their poster "Deep Sleep. A ballistocardiographic-based Deep Learning approach for classifying sleep stages." This poster was selected out of 98 poster of which 12 were nominated.
Authors: Shashank Rao (TU Delft), Abdallah El Ali (CWI), Pablo Cesar (CWI / TU Delft)
Link to presented poster:
ABSTRACT
Current techniques for tracking sleep are either obtrusive (Polysomnography) or noisy with low accuracy (wearables). In this work, we aim to model a sleep classification system using an unobtrusive Ballistocardiographic (BCG)-based heart sensor signal collected from a commercially available pressure-sensitive sensor sheet. We present our early work on our DeepSleep model, a hybrid deep neural network architecture comprising of CNN and LSTM layers, which is able to classify sleep stages with a mean F1-score of 74% using the BCG signal. We further employed a 2-phase training strategy to build a pre-trained model and to tackle the limited dataset size. With a classification accuracy of 83%, 77% and 63% using MIT-BIH’s ECG, Dozee’s ECG and Fitbit’s PPG datasets, respectively, we contribute early results that show promising results in such transfer learning settings. Furthermore, with a correlation coefficient of r = 0.43, our model shows a positive correlation with the SATED questionnaire perceived sleep quality scores. Although our proposed model’s performance is not yet comparable to Polysomnography, we show that heart rate signals alone are a promising means for long-term sleep monitoring.
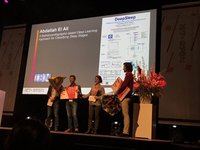