Seminar++ meetings consist of a one-hour lecture building up to an open problem, followed by an hour of brainstorming time. The meeting is intended for interested researchers including PhD students. These meetings are freely accessible without registration. Cookies and tea will be provided in the half-time break.
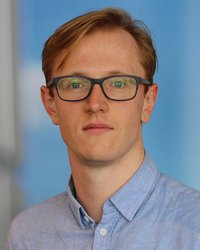
Dirk van der Hoeven
Postdoc at the University of Amsterdam.
High-Probability Risk Bounds via Sequential Predictors
Abstract: Online learning methods yield sequential regret bounds under minimal assumptions and provide in-expectation results in statistical learning. However, despite the seeming advantage of online guarantees over their statistical counterparts, recent findings indicate that in many important cases, regret bounds may not guarantee high probability risk bounds. In this work we show that online to batch conversions applied to general online learning algorithms are more powerful than previously thought. Via a new second-order correction to the loss function, we obtain sharp high-probability risk bounds for many classical statistical problems, such as model selection aggregation, linear regression, logistic regression, and—more generally—conditional density estimation. Our analysis relies on the fact that many online learning algorithms are improper, as they are not restricted to use predictors from a given reference class. The improper nature enables significant improvements in the dependencies on various problem parameters. In the context of statistical aggregation of finite families, we provide a simple estimator achieving the optimal rate of aggregation in the model selection aggregation setup with general exp-concave losses.
First open problem: This open problem will be the main focus of the seminar. The result for logistic regression is nearly optimal and the algorithm is computationally efficient in the sense that the runtime is polynomial in the relevant problem parameters. However, it is a polynomial with a very high degree, making the algorithm practically not very useful for reasonable problem parameters. For in expectation guarantees it is known how to reduce the runtime to d2 T at the cost of a slightly worse excess risk bound, where d is the dimension of the problem and T is the number of datapoints. Unfortunately it is not immediately clear how to use the ideas from the faster algorithm to obtain a high-probability excess risk bound with a d2 T runtime algorithm. This open problem asks for the following: can we obtain a reasonable excess risk bound with high-probability in d2T runtime for logistic regression.
Second open problem: Our results heavily rely on a particular inequality for exp-concave losses. I would like to extend our ideas to a different class of loss function, namely self-concordant loss functions. Given previous results in statistical learning literature (see https://arxiv.org/pdf/2105.08866.pdf), I expect this to be possible.
Series of 8 meetings: