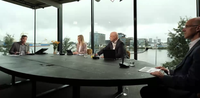
On Monday 13 September 2021, the 23rd edition of CWI in Business took place, this year in cooperation with TNO. Subject this year: Secure Multiparty Computation. Six speakers took the online audience from the scientific foundations to practical applications in, among others, healthcare, fighting financial crime and machine learning.
Imagine having an auction where you want to reveal the winner but keep all the bids secret, even from the auctioneer. Or imagine hospitals that want to cooperate to improve the care for a certain group of patients based on all the available data, but that don’t want to reveal their own data to the other hospitals, for the privacy of their patients.
These are two examples where you want to compute with input data of different parties without each party revealing its own data to another party. What is needed to solve such problems, is a branch of cryptology called secure multiparty computation (MPC). During CWI in Business 2021 CWI researchers Ronald Cramer and Lisa Kohl introduced what MPC is and how it works on the mathematical level.
“In classical cryptography you have to protect the good guys from bad guys who are outside the system”, Cramer told. “That is uni-lateral security. Secure multiparty computation, however, is multi-lateral: the bad guys can be inside the system. There can be mutual mistrust or conflicting interests between various parties.”
From theory to practice
CWI’s Lisa Kohl explained how MPC got its theoretical foundation in the 1980s and how CWI got involved early on. Since 2008, MPC has been applied on a small scale, for instance in auctions of sugar beets and electricity, in voting procedures and in the analysis of statistical data.
Kohl: “Since the eighties, a lot of progress has been made. For a long time MPC was a theoretical promise, but now we can really do things. However, for large scale practical applications there is still a lot to be done.” Cramer added: “And for this, we need an interaction between theory and practice, such as the one we are having with TNO.”
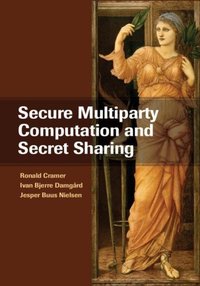
Cover of the handbook 'Secure Multiparty Computation and Secret Sharing' by Ronald Cramer, Ivan Damgård, Jesper Nielsen. Cambridge University Press (July 2015)
Multiparty computation for machine learning
From Sydney, Australia, senior researcher Marcel Keller joined the online event. He works at CSIRO’s Data61 where he has a strong interest in using MPC for privacy-preserving machine learning. Keller: “The idea is that data owners share their inputs among the computing parties. Next, the computing parties train a machine learning model securely using MPC. Finally, they output a model, or use it for secure inference.” Keller showed that that algorithms used in machine learning can be very suitable for applying MPC. Thijs Veugen (TNO and CWI) reacted on Keller’s results: “Showing that even complicated algorithms like a neural network are possible with MPC is a good accomplishment.”
In his own presentation Veugen told the audience about the paradox of two societal trends: on the one hand combining data can lead to a lot of value creation, but on the other hand regulations like the European GDPR require privacy and confidentiality of data. Veugen showed how MPC can help banks cooperating in fighting money laundering without sharing their data with the other banks. The second case he explained deals with finding overlapping items in two databases owned by two different parties who don’t want to share their data with the other party, for example data about pensions by Sociale Verzekeringsbank (SVB) and data about incomes from Uitvoeringsinstituut Werknemersverzekeringen (UWV).
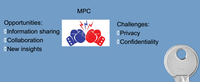
How multiparty computation can improve healthcare
PhD-student at CWI Mark Abspoel showed the results of research he did back in 2017 and 2018 on improving HIV treatment choice by MPC. HIV treatment involves two types of data that are sensitive. For liability reasons, doctors are not keen to publish their decisions on which specific treatment they had chosen for which patient at which moment in time. Patients, from their side, want that the genotype of their HIV-infection stays private as it might contain sensitive information. Abspoel: “We have shown that MPC enables new solutions to this problem and also that the performance can be good enough for practical application.”
Final speaker Martine van de Gaar introduced the TNO spin-off company Linksight that she co-founded in 2021. Linksight applied MPC for the first time in the Netherlands on real patient data. Van de Gaar: “We investigated what happens with the health care provided by general practitioners, by pharmacies and by hospitals when patients with a chronic illness are using an E-health app. An important lesson learned can be summarized as: ‘no real data means no real learning’. Working with real data forces you to think about the real world legal, security and business aspects, and about the people described by the data.”
More information
- The CWI in Business 2021 presentations are available here.
- If you missed the webinar, you can view CWI in Business 2021 here.
- CWI in Business 2021 was organized by Prof. dr. Ronald Cramer (CWI, UL) – head of CWI’s Cryptology group - and dr. ir. Thijs Veugen (TNO and CWI).