Evolutionary Algorithms (EAs) have proven to be effective tools for addressing various optimization challenges, drawing inspiration from natural selection and offspring creation processes. Arkadiy provides an in-depth exploration of the Gene-pool Optimal Mixing Evolutionary Algorithm (GOMEA) within the context of diverse optimization problems, including real-world ones. GOMEA's distinctive model-based approach exploits problem-specific information to streamline the optimization process, capitalizing on existing variable dependencies.
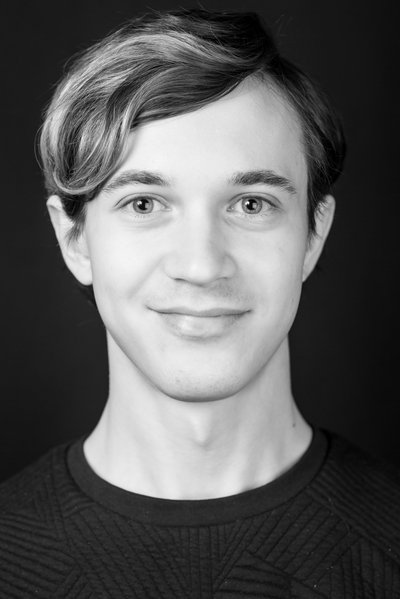
In his thesis Arkadiy concentrates on a special type of optimization: problems for which calculating the objective value of a solution is computationally expensive. Hence, it is advantageous to replace this calculation with a surrogate estimation—a computationally inexpensive yet imperfect approximation of the objective value made by a fitted surrogate model. The researcher integrates this surrogate modeling technique into GOMEA and demonstrates that the resulting algorithm outperforms the existing alternatives.
Reduce workload of clinicians
He also proposes a novel approach to medical image segmentation (identifying and contouring organs in medical scans, like MRIs) which holds potential for greater applicability and better reception in a clinic. Its main idea is to automatically produce several plausible variants of segmentation. When presented to a clinician, Arkadiy states, there is a high chance that at least one variant will be deemed acceptable or require minimal manual correction. “To achieve this, we combine Deep Learning (a standard modern approach to medical image segmentation) with the developed efficient Evolutionary Algorithms. In this context, they are used to identify distinct groups of images in the training data. Subsequently, multiple Deep Learning models can be trained on these groups, resulting in slightly different segmentations.”
Experiments with this method applied to real clinical data demonstrate its potential for practical application. Ultimately, automatic segmentation methods might reduce the workload of clinicians and help to perform diagnostics and treatment faster.
Arkadiy Dushatskiy: ‘Expensive optimization with model-based evolutionary algorithms applied to medical image segmentation using Deep Learning’
- Promotor: Prof. dr. Peter Bosman (CWI / Delft University)
- Co-promotor: Dr. Tanja Alderliesten (Leiden University)